The Role of Annotation in Machine Learning: Enhancing Business Efficiency
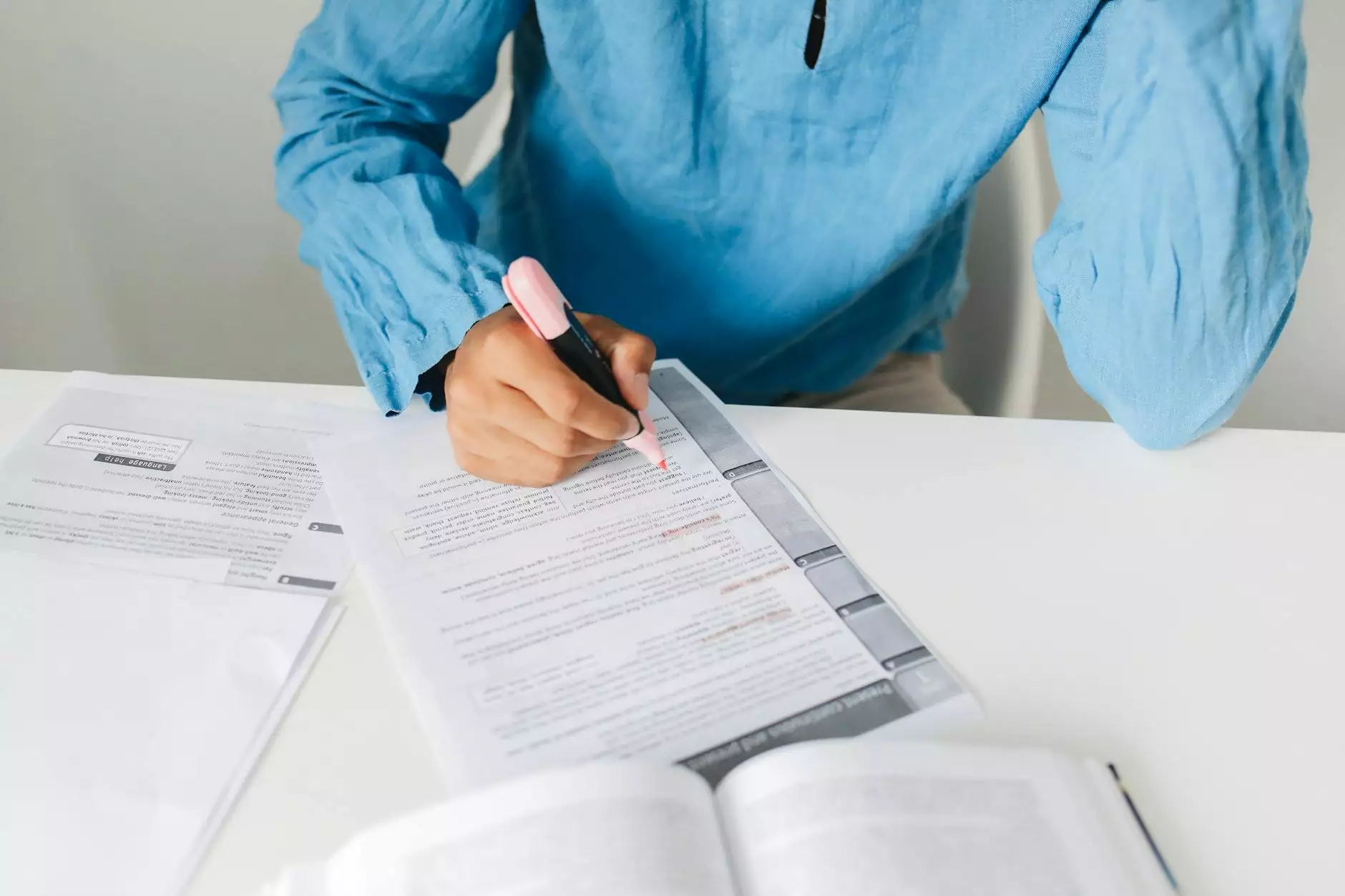
In today's fast-paced world, the integration of machine learning into various sectors is transforming how businesses operate. One core component that drives successful machine learning models is annotation in machine learning. Annotation refers to the process of labeling data to enable algorithms to learn and predict outcomes effectively. In this extensive article, we will explore the significance of annotation within the realm of machine learning and its profound impact on businesses, particularly within the Home Services, Keys, and Locksmiths categories.
Understanding Annotation in Machine Learning
Annotation in machine learning acts as the foundational layer where data is meticulously labeled to guide the learning process of algorithms. This comprehensive procedure is essential for both supervised and unsupervised learning models, as it allows systems to recognize patterns and make predictions based on labeled data.
The Significance of Data Annotation
Data is the lifeblood of machine learning. However, raw data is often unstructured and lacks the necessary context for algorithms to learn effectively. Here’s why annotation is critical:
- Improved Model Accuracy: Labeled data increases the accuracy of predictions by providing clear examples for the model to learn from.
- Facilitates Better Training: Annotation helps in training algorithms with relevant contexts, minimizing errors in data processing.
- Objective Data Analysis: Annotated data enables analysts to extract insights and trends that inform better business strategies.
Types of Annotation in Machine Learning
Various types of data require different methods of annotation, which can be broadly categorized into:
1. Image Annotation
This technique involves labeling images so that a machine learning model can understand the content. Common tasks include object detection, image segmentation, and facial recognition, all of which are crucial for industries like security and home services.
2. Text Annotation
In the context of language processing, text annotation includes labeling parts of speech, sentiment tagging, and named entity recognition. This is particularly valuable for customer service applications, such as chatbots utilized by locksmith businesses to enhance customer experience.
3. Video Annotation
For businesses that incorporate video technologies, video annotation is essential. It involves labeling frames of a video sequence for applications in surveillance, automotive industry, and real estate, where foot traffic can be analyzed to improve service offerings.
Implementing Annotation in Home Services and Locksmiths
Businesses like keymakr.com, which specialize in home services and locksmith solutions, can harness the power of annotation in machine learning to revolutionize their operations. Below are several ways in which annotation can impact their efficiency and service delivery:
Enhancing Customer Interaction
By implementing text annotation in customer service communications, locksmiths can train chatbots to respond intelligently. This reduces response times and enhances customer satisfaction.
Optimizing Inventory Management
In image annotation, businesses can label product images to track inventory more effectively using machine learning algorithms that predict stock levels and optimize supply chains.
Improving Safety Measures
Annotation applied to video surveillance footage allows locksmiths to analyze footage for suspicious activity, providing insights that enhance security protocols.
Benefits of Machine Learning Annotation for Businesses
The adoption of machine learning, supported by effective annotation, presents numerous advantages for businesses, especially in the competitive fields of home services and locksmithing:
- Cost Efficiency: By automating routine tasks, businesses can redirect human resources to more complex problems, thereby reducing costs.
- Data-Driven Decisions: Annotated data enables businesses to make informed decisions by analyzing patterns and customer preferences.
- Faster Service Delivery: Machine learning can predict service demands, allowing locksmiths to prepare and respond more swiftly.
Challenges of Annotation in Machine Learning
While the implications of annotation in machine learning are profound, challenges remain. Understanding these obstacles is vital for businesses aiming to integrate this technology:
Data Quality Issues
Inconsistent or low-quality data can lead to misleading insights. Therefore, businesses must ensure high-quality annotations to achieve reliable outcomes.
Resource Intensive
Manual annotation can be time-consuming and expensive, especially when dealing with large datasets. However, automation and outsourcing can help mitigate these issues.
Need for Continuous Updates
As markets evolve, models must stay relevant, necessitating regular updates and retraining with new annotated data.
Future Trends in Annotation and Machine Learning
As we look towards the future, several trends are likely to reshape the landscape of annotation in machine learning:
1. Automated Annotation Tools
The rise of tools that automate the annotation process will significantly reduce the time and cost associated with data labeling, enabling businesses to leverage machine learning more effectively.
2. Crowdsourcing for Annotations
Crowdsourcing platforms can tap into a vast pool of contributors, allowing for quicker and more diverse annotations, thus enriching data quality.
3. Enhanced Algorithms for Annotation
Developments in algorithms that can learn to annotate data with minimal human intervention are on the horizon, making the processes more efficient.
Conclusion
In conclusion, annotation in machine learning is not just a backend process; it is a game-changer that can propel businesses like keymakr.com to new heights of efficiency and service quality. By investing in robust annotation processes, businesses in the home services and locksmith industries can better understand customer needs, streamline operations, and ultimately enhance profitability. As technology continues to advance, embracing tools and practices that support effective annotation will be imperative for businesses looking to stay competitive in the market.
As you delve deeper into the world of machine learning and its applications, remember that the core component of successful models lies in the quality of data annotation. A well-annotated dataset is your gateway to unlocking the true potential of machine-driven insights and innovation in your field.